Revolutionizing Image Analysis with an Image Segmentation Labeling Tool
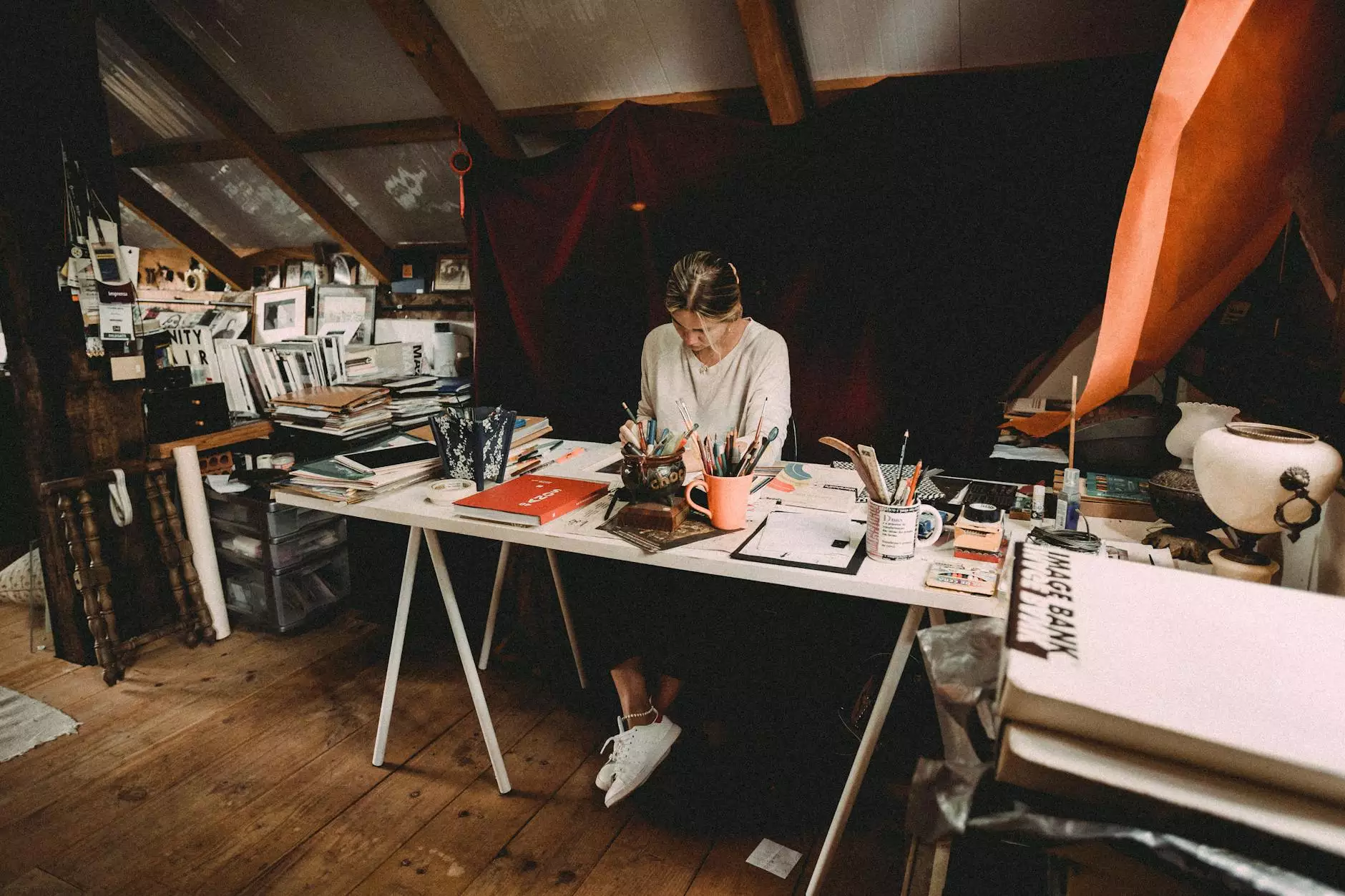
The modern landscape of technology and artificial intelligence is heavily influenced by image recognition and computer vision. To unlock the full potential of these technologies, developers and researchers require tools that enhance their workflows, enabling faster and more accurate results. Enter the image segmentation labeling tool, a game-changer in the realm of dataset preparation.
Understanding Image Segmentation
Before delving into the specifics of an image segmentation labeling tool, it is crucial to understand what image segmentation entails. Image segmentation is the process of partitioning an image into multiple segments, typically to simplify its representation and make it more meaningful for analysis.
Why Image Segmentation Matters
The importance of image segmentation lies in its ability to:
- Simplify Data Analysis: By dividing images into segments, it allows for more straightforward analysis of objects and areas of interest.
- Enhance Object Detection: Facilitating better detection rates and understanding of various entities within an image.
- Support Machine Learning: Providing labeled datasets for training models, thus enhancing their accuracy and efficiency.
The Role of an Image Segmentation Labeling Tool
An image segmentation labeling tool plays a pivotal role in facilitating the segmentation process. It automates and streamlines the labeling of images, allowing for more efficient workflows. Here are key functionalities and benefits of using such tools:
Key Functionalities of an Image Segmentation Labeling Tool
An effective image segmentation labeling tool should offer:
- Interactive Segmentation: Users can manually adjust segment boundaries for accuracy, ensuring high-quality results.
- Automated Labeling: Utilizing AI, these tools can auto-generate labels based on pre-trained models, speeding up the process.
- Customizable Workflows: Adapting to the specific needs of your project, whether for medical imaging, autonomous vehicles, or general object recognition.
- Export Options: Offering various formats for exporting labeled data compatible with different machine learning frameworks.
Enhancing Efficiency and Quality
The efficiency of an image segmentation labeling tool reduces the time taken for dataset preparation significantly. Manually labeling images can be laborious and prone to errors, whereas automated and semi-automated systems ensure consistent quality and reduce human intervention.
The Impact on Software Development
In the realm of software development, especially in projects involving computer vision, the integration of an image segmentation labeling tool can drastically improve turnaround time and project outcomes.
Improved Collaboration and Productivity
By streamlining the labeling process, teams can collaborate more efficiently. Developers, data scientists, and researchers can focus their efforts on innovation rather than getting bogged down in the details of data labeling.
Real-World Applications
The applications of image segmentation are vast and varied:
- Healthcare: Segmentation tools are critical in medical imaging for identifying tumors or anatomical structures.
- Autonomous Driving: Vehicles rely on accurate segmentation for understanding their environment, detecting pedestrians, road signs, and other vehicles.
- Agriculture: Analyzing crops and land health through segmented images aids in better management practices.
Choosing the Right Image Segmentation Labeling Tool
With a myriad of options available, selecting the right image segmentation labeling tool can be daunting. Here are some factors to consider:
Key Selection Criteria
- User-Friendliness: The tool should offer an intuitive interface to minimize the learning curve.
- Feature Set: Look for tools that provide robust features for both manual and automated labeling.
- Integration Capabilities: Ensure compatibility with your existing workflows and tools.
- Support and Community: A strong user community and professional support can greatly enhance your experience.
Case Studies of Successful Implementations
Many organizations have benefited from implementing an image segmentation labeling tool:
Example 1: Healthcare Imaging Solutions
A leading healthcare provider adopted an advanced labeling tool for segmenting MRI scans. This significantly enhanced their diagnostic accuracy and reduced the time required to prepare training datasets from weeks to days.
Example 2: Autonomous Vehicle Development
A startup focusing on autonomous vehicles utilized a segmentation tool to enhance their object detection algorithms, resulting in a marked improvement in the reliability of their self-driving technology.
Future Trends in Image Segmentation Technology
The field of image segmentation is rapidly evolving. Here are some anticipated trends that may shape the future:
Advancements in AI and Machine Learning
As AI technologies continue to develop, we can expect even more sophisticated segmentation tools that learn from user interactions to improve accuracy over time.
Integration with Augmented Reality (AR)
Combining image segmentation with AR could lead to revolutionary applications in shopping, training, and gaming.
Automated Data Annotation
Future tools may leverage deep learning to automate not just segmentation but also data annotation, thereby drastically improving productivity.
Conclusion
In summary, an image segmentation labeling tool is an invaluable asset in the arsenal of developers and researchers working in the fields of machine learning, computer vision, and data science. Its benefits include enhanced data quality, decreased preparation time, and broad applicability across various domains. As technology progresses, the role of these tools will only grow more critical, making it essential for professionals to stay informed and choose the right solution for their specific needs.
For more insights and the latest developments in software solutions, visit keymakr.com, where innovation meets efficiency.